Will your bedsheet kill you if you consume cheese? Aka, does Correlation imply Causation?
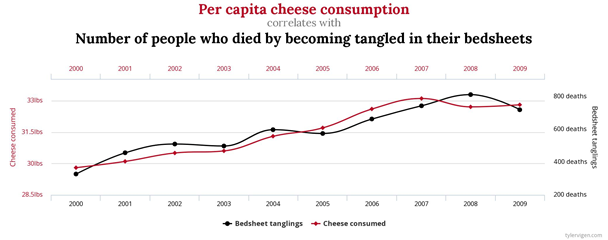
Most probably you are speculating if am too inebriated to make sense on a weekday afternoon. See the above chart.
The resources on Statistical analysis that you have been pondering on would totally agree with this, given the clear positive correlation between the two variables. But, as a Data Science professional, can you convincingly prove that cheese consumption causes death by bedsheet tangling?
This, is one of the most common and noteworthy errors made in statistical analysis.
Correlation Vs Causation
Correlation and Causation are very important Data Science concepts. The two concepts are quite meaningful & powerful in Data inferences on their own. But it can be confusing if not used correctly. Usually Correlation is confused as Causation, which is not the truth.
Ben Yoskovitz, Founding Partner at HighlineBeta, explains the difference between correlation and causation by stating “correlation helps you predict the future, because it gives you an indication of what’s going to happen. Causality lets you change the future.”
Andrew Lang, a Scottish scholar said- “An unsophisticated forecaster uses statistics as a drunken man uses lamp-posts — for support rather than for illumination”. As he rightly puts across, data in the right hands illuminates, but in the wrong hands draws conclusions such as Facebook driving the Greek Debt Crisis, or the baby name ‘Avas’ causing the US housing bubble (Bloomberg).
To draw logical and accurate conclusions from data, one need to know the statistical concepts thoroughly.
According to the Bureau of Statistics correlation is, “A statistical measure (expressed as a number) that describes the size and direction of a relationship between two or more variables.”
While causation “Indicates that one event is the result of the occurrence of the other event; i.e. there is a causal relationship between the two events. This is also referred to as cause and effect.”
The classic causation vs correlation example that is frequently used is that smoking is correlated with alcoholism but doesn’t cause alcoholism. While smoking causes an increase in the risk of developing lung cancer.
For two variables, a statistical correlation is measured using a Correlation Coefficient, represented by the symbol (r), which is a single number that describes the degree of relationship between two variables. The coefficient’s numerical value ranges from +1.0 to –1.0, which provides an indication of the strength and direction of the relationship.
A causal relation between two events exists if the occurrence of the first causes the other. The first event is called the cause and the second event is called the effect. A correlation between two variables does not imply causation. On the other hand, if there is a causal relationship between two variables, they must be correlated.
While Correlation can be calculated, Causation cannot be. Data contains only Correlation and not Causation. Causation is often the way we represent correlation mentally (in our imagination).
Causality has more to do with common sense, experience and clear thinking than with the value of r. Unless controlled intervention is introduced at some point in the data collection, we cannot draw dependable conclusions about causality. To this day, debate continues about whether causation is a feature of the physical world or simply a convenient way to think about relationships between events.
With the emergence of big data enormous data sets are collected automatically. Using this correlations can be mass-produced. The trouble is that many of them will be meaningless. This is known as the problem of “false discovery.” A small number of meaningful associations is easily drowned in a sea of chance findings. Statisticians have developed theories and tools to deal with the problem of chance findings. Perhaps best known is the p-value, which can be used to assess whether an observed association is consistent with chance, or conversely, as it is commonly put, that it is “statistically significant.” To be a successful Data Science Professional, it is imperative to understand the Statistical concepts very well.
Read — https://cran.r-project.org/web/packages/generalCorr/vignettes/generalCorr-vignette.pdffor using R to measure Correlation & Causation.
Explore Our Programs
Institute of Product Leadership is Asia’s First Business School providing accredited degree programs and certification courses exclusively in Product Management, Strategy, and Leadership.
Talk to a counselor today and embark on your journey towards becoming an exceptional product manager.
Whether you’re seeking advice on career paths, looking to enhance your skills, or facing challenges in your current role, our counselors are ready to provide valuable insights and actionable strategies.
Visit IPL’s school of Data Science to master the cutting edge skills of the future