The Rise of the Data Smart Manager
Who are they?
Data Smart Professionals are those who know how to operationalize insights from data to drive businesses and decisions. Data Smart Professionals have a keen interest in driving data driven decision making at various levels in an organization. These Data Smart Professionals are also sometimes called data savvy Professionals. Our definition of Data Smart Manager is one who has a strong business acumen, cross-functional and people management skills, good understanding of data science principles, algorithms, stats, and math.
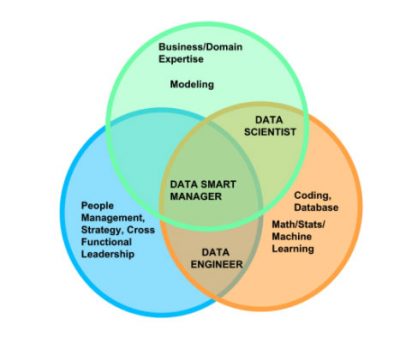
Why are they important?
What skills do they possess?
While there are many skills that Data Smart Professionals possess, here are some important ones:
Asking the right questions: Data Smart Professionals ask the right questions. Scientific research is based on either inductive or deductive logic. Deductive logic starts with a premise, collects data and then draws conclusions. The opposite of deductive logic is inductive logic, where the sequence is that first you have data, and then you make your conclusions from specific to general. Thanks to the data revolution, we now have huge datasets and there is a need to find patterns in them. In a sense this is a journey of finding questions based on the answers that are hidden in data.
Design of experiments: There is a difference between analytics and experimentation. Professionals involved in business decisions should increasingly rely on ‘test and learn’ than mere intuition. For analytics to succeed the right framing of problem is critical. The first step to problem framing is asking the right questions. The second is to design the right business experiment. For example A/B testing is one of the most effective ways of finding out which version of the website is working best. We need such type of test and learn experiments for data science problems as well.
Understanding of Algorithms, Stats and Math: A basic understanding of data science techniques and underlying Stats and Math is a must. A Data Smart Manager may not know how Eigenvalue is calculated, but should know Eigenvalue could help in determining the ideal number of clusters.
Data Evangelism and Leadership: It is also critical that Data Smart Professionals ensure the operationalizing of data driven decision making into the nitty gritty of an organization’s working. A Data Smart Manager needs to drive data driven decision making with an end goal in mind.
How can you become a Data Smart Manager?
There are two ways in which this quant crunch can be managed. One is to make existing domain experts more data savvy by training them on data skills and the interlock between data and business. The other way is to train people on both skills.
This article appeared first in the Silicon India Magazine